Workshop #3
EDA for AI, and AI for EDA
"Machine Learning for Microfluidic Design and Automation"
Prof. Tsung-Yi HO (CUHK)
Abstract
Microfluidics or Lab on a Chip (LoC) system provides a powerful integrated platform for automated manipulation of different solutes, droplets, particles or even tissue at the microscale. Unlike conventional microfluidic devices, current LoC systems usually have multiple units with various functionalities, which requires microfluidic-oriented design automation tools to facilitate researchers during the design process. To address this issue, machine learning techniques might be a promising solution for microfluidics/LoC design automation with its ability to learn from both experimental data and numerical data. In this talk, different aspects related to this field will be presented and discussed.
Reference
- Liang et al., Adaptive Droplet Routing in Digital Microfluidic Biochips Using Deep Reinforcement Learning, ICML'20.
- Liang et al., Parallel Droplet Control in MEDA Biochips using Multi-Agent Reinforcement Learning, ICML'21.
- Wang et al., Predicting the fluid behavior of random microfluidic mixers using convolutional neural networks", LoC'21
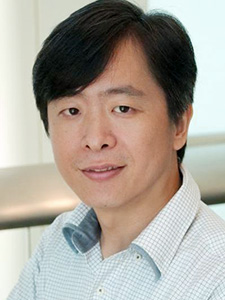
Prof. Tsung-Yi HO
"VLSI Mask Optimization: From Shallow To Deep Learning"
Prof. Bei YU (CUHK)
Abstract
The continued scaling of integrated circuit technologies, along with the increased design complexity, has exacerbated the challenges associated with manufacturability and yield. In today’s semiconductor manufacturing, lithography plays a fundamental role in printing design patterns on silicon. However, the growing complexity and variation of the manufacturing process have tremendously increased the lithography modeling and simulation cost. Both the role and the cost of mask optimization – now indispensable in the design process – have increased. Parallel to these developments are the recent advancements in machine learning which have provided a far-reaching data-driven perspective for problem solving. In this talk, we shed light on the recent deep learning based approaches that have provided a new lens to examine traditional mask optimization challenges. We present hotspot detection techniques, leveraging advanced learning paradigms, which have demonstrated unprecedented efficiency. Moreover, we demonstrate the role deep learning can play in optical proximity correction (OPC) by presenting its successful application in our full-stack mask optimization framework.
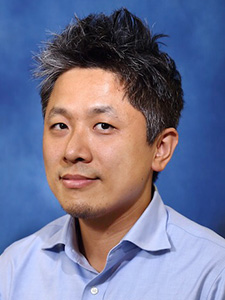
Prof. Bei YU
"Exploring AI-assisted Optimization Opportunities in Placement and Routing"
Prof. Yibo LIN (PKU)
Abstract
Placement and routing are critical stages in the IC design flow. Solving placement and routing involves many optimization problems, which are computationally expensive or hard to even formulate the objectives. Recent boom of deep learning techniques brings new opportunities to tackle these optimization problems from various perspectives. In this talk, we will introduce recent progress in deep learning assisted optimization in placement and routing, and show case studies on how deep learning helps cross-layer optimization with accurate modeling and automatic strategy search.
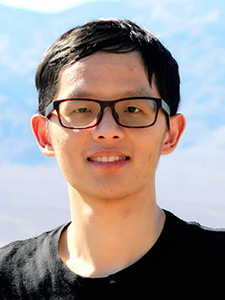
Prof. Yibo LIN