Public Lecture #1
"Emerging Memory Enabled and Heterogeneously Integrated AI Chips and Systems”
Prof. Tim CHENG (HKUST)
Abstract
We will give an overview of some recent results on 3D integration of CMOS and memristive memory arrays and demonstrate its potential of offering very high memory density and bandwidth at manageable power dissipation, and enabling new memory-centric computing paradigms for AI applications.
It has been recognized that such resistive memory cells still suffer from multiple limitations including high energy consumption, limited endurance, and large cycle-to-cycle and device-to-device variations. In this talk, we will highlight some recent solutions addressing these limitations.
Finally, we will present the concept and mechanism of ratio-based encoding for resistive memory cells, in which the resistance ratio of a pair of resistance-switching devices, rather than the resistance of a single device (i.e. resistance-based encoding), is used for encoding the information, which significantly reduces the bit error probability. Applicable to both single-level and multi-level memory cells, we demonstrate the advantages of the ratio-based encoding over the resistance-based encoding for designing robust resistive memory systems. We show that, compared to the resistance-based encoding on the same resistive devices, the proposed approach achieves up to 3 orders of magnitude lower bit error probability, or alternatively it could reduce the cell’s programming time and programming energy by 5–10X , while achieving the same bit error probability.
References
- M. Lastras-Monta, O.D. Pozo-Zamudio, L. Glebsky, M. Zhao, H. Wu, and K.-T. Cheng, “Ratio-based Multi-level Resistive Memory Cells”, in Scientific Reports, January 2021.
- M. Angel Lastras-Montano, and K. -T. Cheng, "Resistive Random-access Memory based on Ratioed Memristors", Nature Electronics, 2018.
- K.-T. Cheng, N. Gong, and M. A. Lastras-Montaño, "Variability in emerging memory devices and solutions," in Section 8 of Roadmap paper Roadmap on Emerging Hardware and Technology for Machine Learning, Qiangfei Xia et al., Nanotechnology, January 2021.
- http://vsdl.ust.hk/
- https://seng.ust.hk/about/people/faculty/tim-kwang-ting-cheng
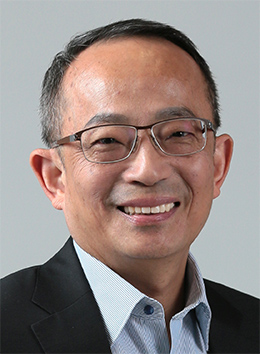
Prof. Tim CHENG